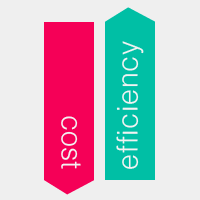
In the previous part we noticed that if we reduce premium and costs with the same absolute value, we get a better result at the end of the period, ceteris paribus.
It would indeed be interesting to know the reason to reach this surprising result. To work it out, we need to look at more theoretical aspect of our model. If we parameterize the variables, we can trace in depth the changes and differences that occur in each of them in development of the two parallel scenarios.
We introduce the following parameterization of the model:
Let us express the parameters of one insurance policy at time t in accordance with the development of the first scenario. The price of a single policy is P, with acquisition costs a percent of premium and administrative expenses d percent of premium. The value of the UPR is proportional to the premium – let us denote it by k (t) * P, where k (t) ε [0, 1]. The coefficient k (t) indicates how much of the premium of the policy is unearned at the moment t. So let’s look at the values of the two parallel scenarios at time t from the beginning of the policy. For the first one we have:
(6)
Consider these variables under the assumption of reducing the acquisition costs by Δa percent of P and administrative expenses by Δd percent of P. Let the reduction of these expenses be a “gift” for the customer. This means that the cost of the policy would be P * (100-Δa-Δd)% and analogously to (4), adjusted percentages of the costs of the new premium would be:
In this situation the considered variables have the following expression at time t :
Let us now compare the results of realization of both scenarios. According to (3) and (6) we have:
and for the second one we have:
So, for the difference between the two results we get:
(7)
A really curious result has occurred! It turns out that the difference in the development of the two scenarios is due to lower administrative expenses, and that if the policy is active at the time of calculation of the result or more precisely, while there is still some unearned premium left over the policy. When the contract expires, all the premium is won then k(t_end)=0 and the impact of this particular insurance policy on the overall result becomes equal in both scenarios. Let us go back to played above two scenarios in which we measured and compared the results at the end of the financial year. Just for verification, we apply the result (7) on the above considered example. According to findings made so far, we easily consider the following:
And substituting with the results already obtained in (2) and (5) we get:
The application of (7) in this case has the following expression:
i.e.,
,
which confirms the conclusions so far.
In fact, we proved that the difference in the development of the two scenarios is due to the reduced levels of administrative expenses which result in lower values of the difference {UPR-DAC}.
It turned out that “giving” money from reduced costs to the end consumer, does not lead to a weaker result, but even improve it within a year. And with that good news does not end. Maintaining lower prices and observing the development in the second scenario , we did not take into account one key economic indicator – the price elasticity of demand. It is logical to assume in our example that the reducing of the price of policies by 7% would lead to increased demand. And, although consumers would react far more quickly to changes in the price, let us assume that this happens at the beginning of the second year (y2). Assume that in the second year of the development of the second scenario, while maintaining the same price and costs, the demand for MTPL insurance policies increases flatly every day, the level of daily sales increases by three units up to 96 units a day. From the standpoint of economic theory this is relatively inelastic demand, as for the ratio relates the change in the quantity demanded to the price change we have:
In terms of our model, this means that second year ends with the following values of the variables:
So the result formed by these parameters is expressed by the formula:
Substituting in the formula we get:
,
Which is growth by 437.7 units compared to the development in the first scenario, as already shown that:
In the report “European Motor Insurance Markets” is taken notice of the fact that “MTPL policies are somewhat homogeneous across the EU in terms of the cover provided”. According to the authors of the report “In light of the high level of homogeneity in MTPL cover provided in individual member states, competition between insurers is mainly based on pricing and quality of services, rather than on product design”. Fortunately, we chose exactly this product to implement our advanced online platform, which will allow us to keep lower price levels and higher quality of services. Considering this relative standardization of the MTPL products in EU we should expect higher sensitivity of consumers to price, therefore we can calculate results under normal elastic demand:
In our case this means that the decrease in price by 7% in the first year led to increased sales by 7% in the second year, which is equivalent to the realization of daily premium income of 6.51 units more than the daily premium income in the previous year. So, we calculate the values at level of 99.51 units of daily sales:
For the result we obtain:
Here we notice a remarkable difference of 948 units more in the second scenario, which is increase in the technical result by 3.36% compared to the development in the first scenario.
We can conclude that under normal elastic demand and relative elastic demand, while reducing operating costs and prices of policies with the same values, a significant growth in technical results can be expected, ceteris paribus.
In the final part we are going to examine another scenario to represent a different strategy for investing the savings from the reduced costs. We will show the effect of keeping the gained 2% savings of the reduced administrative expenses.
1 Numbers may not add up due to rounding.