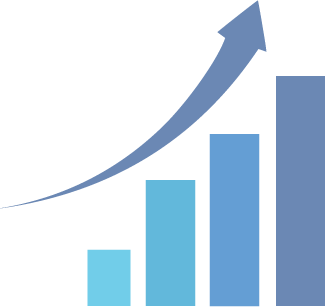
Part 3
In the previous part we studied the reasons for the better development of the second scenario, compared to the first one. We also showed the further development of the second one under the assumptions of relatively inelastic demand and normal elastic demand. In this final part, we observe the development of another, a third scenario, just for comparison. The goal is to measure the result if we decide to keep the savings from the administrative expenses reduction for other projects of the company.
Now we do a little change in pricing policy – we return to the starting point of the year first (y1), when successfully started new online platform, which reduces acquisition costs by 5% and administrative expenses by 2% at daily level of sales of 100 units. Let us again “give” the saved acquisition costs to the end consumer, but keep saved administrative expenses in the company. That decision may be motivated by the ability to finance investments needed for to the implementation of new technology, namely with the funds saved from reduced administrative expenses.
The above assumptions would have the following impact on the model: Every day of the year (y1) sales of 95 units are realized in the following restated relative levels of expenses:
We note that the change in administrative expenses would affect only the amount of administrative expenses themselves, because this part of savings has no impact on the premium, but remain in the insurance company.
We compute the parameters’ values in the approved manner:
Respectively for the technical result we get:
,
which is by 730 units more than the result of the first scenario and 366 units more than the result of the second scenario
. It can be seen how serious effect on the result has a 2% reduction in administrative expenses. It would be interesting to see what will be the effect on further development of the third scenario, as here also can expect sales growth due to the lowering of the price. We leave the reader the opportunity to follow this development in various hypotheses about price elasticity of demand, using the foregoing reasonings and formulations.
Let us again parameterize the model to follow what caused the observed difference. For this purpose, we will consider again the impact of changes on the values of the parameters. We use already displayed in (6) description of development in the first scenario. In this case we also reduce acquisition rate by Δa percent and administrative expenses by Δd percent. But let us now “present” to the client only saved acquisition costs and savings of administrative expenses keep in the company. This means that the price of the policy will be P * (100-Δa)%, and adjusted percentages of the costs of the new premium will be:
At the moment t we have the following state:
And for the result we have:
For the difference between the 3rd and the 1st scenarios we obtain:
This means that upon the transition from development in the first scenario to development in the third scenario for each policy sold, the technical result increases immediately with all the savings of administrative expenses. We do verify with the already calculated data:
,
then
As we noticed, the third scenario showed a higher result than the second one (there is a difference of 366 units more in the third one), which might be expected, given the fact that the savings from the reduced administrative expenses remain in the company under the same sales levels. Here the question is rather to track out in the longer term if the second scenario “overtakes” the third one in a normal elastic demand and relatively elastic demand, but as we said, we leave the reader to do this. Let us make a comparison of the results in the third and second scenarios. We have already parameterized these two results so we easily displayed:
This shows that every day since the beginning of the policy, gap increases, and when the policy expires, then k(t_end )=0, and all of the saved administrative expenses go to the technical result. Again, we can verify with the calculated data. As noted above, we have the following:
,
i.e.
.
Taking
we get:
.
So, we can conclude that our basic model clearly shows that the market behavior associated with the maintenance of lower prices and lower costs is more efficient than one with higher prices and costs. In the above assumptions, we showed a developing scenario of online based MTPL sales, where we reduced prices and costs with the same value and at a subsequent measurement we observed an improved technical result, ceteris paribus. More and more analysis of the insurance market, pay attention to the necessity and beneficial effect of the use of latest achievements in information technology, including systems for management of business processes and risk assessment, online platforms for realization of sales and such for making more direct contact with consumers of insurance services. In this regard, the industry has a lot to “catch up” compared with other areas of social relations. The huge potential to reduce costs and attract new customers with proper risk assessment seems not yet fully realized. Undoubtedly, those who make use of all this would have a good competitive advantage.
The above-mentioned report by Morgan Stanley and BCG pays attention to the forecast that the digitalization of the insurance process would lead to improved results in claims through methods of better risk assessment and limitation of insurance fraud, for example. In our assumptions the possibility of reducing the costs of damages is not taken into consideration, so this may be the subject of a further research.
1 Numbers may not add up due to rounding.